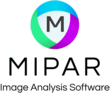

Catalog excerpts
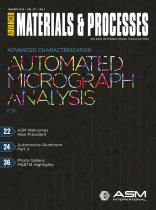
ADVANCED CHARACTERIZATION Automotive Aluminum Part V Photo Gallery: MS&T18 Highlights
Open the catalog to page 1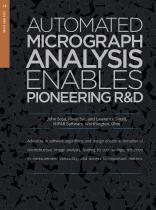
John Sosa, Pavel Sul, and Lawrence Small, MIPAR Software, Worthington, Ohio Advances in software algorithms and design enable automation of microstructure image analysis, leading to cost savings, reduction in measurement variability, and access to important metrics.
Open the catalog to page 2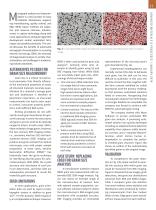
ALTERNATIVES TO EBSD FOR GRAIN SIZE MEASUREMENT Grain size is a critical microstructural parameter that directly influences the mechanical properties of nearly all structural materials. Accurate quantification of a material’s average grain size and distribution is therefore of paramount importance, as inaccurate measurements can lead to poor quality control, inaccurate property predictions, and inefficient R&D cycles. Etching procedures do not sufficiently reveal grain boundaries for optical microscopy in some microstructures and grains are too small to be optically imaged in others. In...
Open the catalog to page 3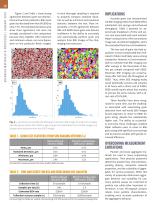
18 A more thorough sampling is required to properly compare standard deviation as well as minimum and maximum statistics between the two. Most importantly, a 97.3% agreement between BSE and EBSD mean grain size delivers confidence in the ability to accurately and automatically perform grain size analysis from BSE images of the challenging microstructure. Figure 2 and Table 1 show strong agreement between grain size distributions and summary statistics. BSE mean grain size deviated from that of EBSD by only 2.7%. The standard deviations also show close agreement, but were not strongly...
Open the catalog to page 4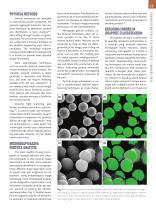
Several techniques are available to characterize particle properties. For granular aggregate materials, the simplest technique to determine particle size distribution is sieve analysis[4,5]. After sifting through meshes of graduated sizes, the percent-by-mass of each size range and a fineness modulus of the material trapped by each sieve is calculated. The technique requires moving samples offline for analysis and it is unable to provide more detailed shape information. More sophisticated techniques such as acoustic emission avoid some of the limitations of sieve analysis. For example,...
Open the catalog to page 5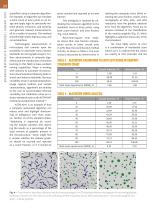
20 quantified using a computer algorithm. For example, an algorithm can simulate a point count at every point on an image and apply logic to subclassify material phases, inclusions, and porosity, and generate relevant measurements, all in a matter of seconds. This method provides high speed, high accuracy, and correctable bias. Technological advancements in microscopes and cameras open the possibility to automate many industry standards for micrograph analysis. Further, advancements in computer algorithms and the introduction of machine learning to this field increase problem solving...
Open the catalog to page 6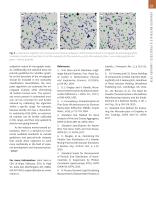
Fig. 5 — Area fraction algorithm error analysis results showing ambiguity in ASTM standard reference micrographs for estimating graphite nodularity in ductile iron (see Table 4): (a) 20% nodularity; (b) 30% nodularity; and (c) 40% nodularity (blue = non-nodular graphite and green = nodular graphite). subjective nature of micrograph analysis. Additionally, the standard does not provide guidelines for whether graphite on the boundary of the micrograph should be included in the nodularity classification. Nevertheless, the computer algorithm can automate the micrograph analysis while...
Open the catalog to page 7All MIPAR catalogs and technical brochures
-
Porosity Analysis
2 Pages
-
Cracks and Pores
2 Pages
-
Aerial Applications
2 Pages
-
Fibers Analysis
1 Pages
-
Fiber Cross Sections
2 Pages
-
Coarse Fibers
2 Pages
-
Satellite Particles
2 Pages
-
Powder Particles
2 Pages
-
Particle Sorting in Asphalt
1 Pages
-
Crystals
2 Pages
-
Cracks in Particles
2 Pages
-
Ti Alloy Microstructure
1 Pages
-
Gamma Prime Detection
1 Pages
-
Alpha Beta in Ti
1 Pages
-
Twin Grains Analysis
1 Pages
-
Grains Analysis
1 Pages
-
Largest Grain
1 Pages
-
Ceramics Grains 2
2 Pages
-
Ceramic Grains
1 Pages
-
Beta Grains in Ti
1 Pages
-
MIPAR API
2 Pages
-
Additive Manufacturing
1 Pages
-
Metals infographic
1 Pages
-
Ceramics infographic
1 Pages
-
Battery Flyer
1 Pages
-
Banner 2
1 Pages
-
Banner 1
1 Pages
-
MIPAR LIVE BROCHURE
4 Pages
-
MIPAR - QAQC Brochure
12 Pages
-
MIPAR Brochure
6 Pages
Archived catalogs
-
Nanoparticles
2 Pages
-
Deep Learning
1 Pages